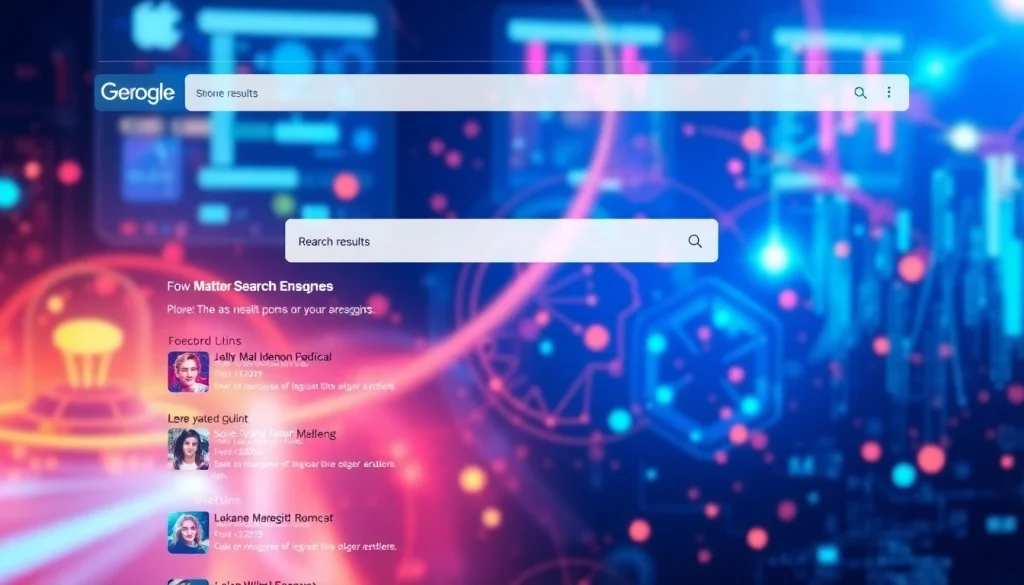
Understanding AI Search Engines
Definition and Key Features
AI search engines are an evolution of traditional search algorithms, integrating advanced artificial intelligence technologies to enhance user experience and information retrieval. Unlike conventional search engines that primarily rely on keyword matching and basic ranking algorithms, AI search engines leverage machine learning, natural language processing (NLP), and data analytics to interpret user intent and deliver more contextual, precise results. They utilize vast datasets to train models, allowing these systems to understand and anticipate user needs.
Key features of AI search engines include conversational interfaces, real-time data analysis, and context-aware search capabilities. For instance, they can interpret complex queries in natural language, providing more than just links to web pages; they generate comprehensive, synthesized answers to user questions. An AI search engine can also personalize results based on the user’s search history, preferences, and current trends, creating a more user-centered experience.
How AI Enhances Traditional Searching
The integration of AI into search technology addresses several limitations of traditional search methods. One important enhancement is the ability to understand context and nuances in queries. For example, a user searching for “best places to eat” can receive tailored recommendations based on previous dining preferences or current location, rather than generic results.
Moreover, AI search engines can continually improve through user interaction. By analyzing feedback and click-through rates, these systems learn which types of information are most relevant to users, refining their algorithms for better accuracy over time. This dynamic learning capability allows them to adapt to changing user preferences and emerging topics, ensuring that search results remain fresh and relevant.
Popular AI Search Engines Overview
Several AI search engines have emerged, each with unique features and strengths. Among them:
- Google’s Gemini: Google has incorporated AI into its search offerings through Gemini, a platform that not only supports conventional searches but also adds conversational capabilities for more contextual help.
- Perplexity AI: Known for its capacity to combine generative AI with real-time web search, Perplexity excels in delivering structured answers while citing sources, allowing users to verify information easily.
- Bing AI: Microsoft’s Bing has also embraced AI, providing integrated features such as Copilot which act as helpful assistants throughout the search process.
- iAsk: A free AI search engine that specializes in educational contexts, allowing users to ask specific questions and receive direct, concise answers, particularly useful for academic needs.
- Consensus: Designed for academic research, Consensus uses AI to sift through vast amounts of academic literature, providing instant insights into complex topics.
Benefits of Using AI Search Engines
Improved Accuracy in Results
One of the compelling benefits of AI search engines is the enhanced accuracy in results. Traditional search engines often return multiple links, requiring users to sift through them to find relevant information. In contrast, AI-driven platforms assess user intent and context to present information that is most likely to meet the searcher’s needs.
For instance, if a user queries something based on recent news, an AI search engine can prioritize content from credible news sources published within the last 24 hours, significantly increasing the relevance of the results. This capability reduces information overload and allows users to find valuable content more efficiently, which is essential in our fast-paced digital environment.
Faster Information Retrieval
Time is often of the essence in information retrieval, particularly in dynamic fields such as technology, finance, and medicine. AI search engines utilize advanced algorithms and databases to deliver answers swiftly, often instantaneously. By processing millions of data points in real-time, these engines make it possible for users to receive quick, reliable answers without scrolling through endless pages of search results.
Additionally, AI search engines streamline the search process by identifying the most pertinent content and surfacing it at the top of the results list. This efficiency not only aids individual productivity but also enhances decision-making across organizations by providing timely access to critical information.
Personalized User Experience
Personalization is another significant advantage offered by AI search engines. These platforms can track individual user behavior and preferences, tailoring search results to align with previous interactions. For example, a returning user who frequently searches for technology news will receive more technology-focused results, whereas a user interested in health topics will see top articles related to medicine and wellness.
By creating a tailored search experience, users feel a deeper connection to the technology, enhancing usability and satisfaction. Furthermore, this personalization can evolve over time, continuously refining the user experience based on new data and interaction patterns.
Key Comparisons with Traditional Search Engines
Speed vs. Relevance
While speed has always been a crucial factor in search engine performance, the introduction of AI has started to tip the scales towards relevance as well. Traditional search engines prioritize speed, often returning results almost instantaneously, but these results do not always meet the user’s needs. AI search engines improve upon this by not only providing fast responses but also ensuring that the information is highly relevant to the query posed, leading to a more satisfactory user experience.
User Interaction Enhancements
AI search engines are characterized by their enhanced user interaction capabilities. Features like conversational AI, voice search, and dynamic suggestions allow users to engage with the search engine in intuitive ways. Unlike traditional search engines which require specific keywords or phrases, AI systems can understand natural language and contextual nuances, making them easier for a broader audience to use.
In addition, many AI search engines can learn from these interactions to improve future searches, creating an iterative loop of enhancement that traditional search engines cannot replicate. This enhanced interaction not only brings greater satisfaction but also improves efficiency, as users can convey complex requests without having to rephrase or simplify their queries.
Data Processing Techniques
AI search engines utilize advanced data processing techniques, such as machine learning and neural networks, to analyze vast amounts of unstructured data. This approach contrasts sharply with traditional search engines that primarily rely on keyword density and basic indexing strategies. Through deep learning methods, AI engines can recognize patterns and relationships within data, offering insights and context beyond the raw information.
For example, they can dissect the underlying trends in user behavior across various demographics, allowing them to predict what information users are likely to seek in the future. This foresight diminishes the lag typical of traditional search engines that must wait for user queries to understand what information is in demand.
Challenges and Considerations
Privacy Issues
The integration of AI into search engine technology raises significant privacy concerns. As these engines require extensive data collection to improve personalization and accuracy, users may be reluctant to share personal information due to fear of surveillance and data misuse. Ensuring robust data protection and transparency will be vital for AI search engines to gain user trust and acceptance.
Organizations employing AI search solutions must adhere to stringent privacy regulations and implement measures to safeguard sensitive user data. This may include anonymizing data, providing options for users to manage their data sharing preferences, and ensuring compliance with legislation such as GDPR.
Dependence on Data
The effectiveness of AI search engines heavily relies on the availability and quality of data. Poorly curated or biased datasets can lead to inaccurate search results, which can compromise user trust. There is also a dependency on continuous data updates to ensure accuracy; without fresh information, these engines can provide outdated results, undermining their utility.
Search engines must establish strong data governance practices, ensuring data quality and diversity to enhance the accuracy of results. Furthermore, they need to implement algorithms that can intelligently filter or rank information to address potential biases in the data.
Accuracy vs. Misinformation
With the proliferation of misinformation online, AI search engines face the challenging task of distinguishing reliable sources from false or misleading information. While AI can analyze the credibility of sources based on specific metrics, it can also inadvertently propagate false information if not carefully monitored. Critical examination of algorithms and consistent updates to verification processes are necessary to reduce misinformation risks.
To combat misinformation, AI search engines can employ mechanisms for cross-referencing sources, prioritizing content from reputable publishers, and allowing user feedback to flag questionable content. This creates an additional layer of reliability, reassuring users that the information they are accessing has undergone thorough vetting.
The Future of Search Technology
Trends in AI Development
The future of AI search engines is set to be transformed by several emerging trends. One significant trend is the evolution of multimodal AI, which integrates various types of data—text, images, and voice. This technology will enable users to search using different content types, expanding the potential for intuitive interactions.
Additionally, the continued development of generative AI will refine how information is presented and summarized. Future AI search engines may evolve into fully-fledged digital assistants capable of conducting broader tasks, such as providing summaries of complex topics or managing schedules based on user inquiries.
Predictions for Next-Gen Search Engines
As the landscape of search technology evolves, next-gen search engines will likely offer augmented reality (AR) and virtual reality (VR) capabilities, allowing users to engage in immersive search experiences. For example, users might visualize historical site tours based on searches, which would lead to more engaging and informative encounters.
Also, ethical AI will gain prominence; ensuring that AI search engines prioritize user welfare and actively work to mitigate issues like algorithmic bias will become a competitive differentiator. Companies that invest in socially responsible AI will be better positioned as user consciousness around these issues grows.
The Role of User Feedback
User feedback will be critical in shaping the evolution of AI search engines. Engaging users in dialogues about their experiences, preferences, and challenges allows developers to refine algorithms and improve user experiences continually. Feedback loops can include ratings, reviews, and more quantitative interaction data. This continual learning mechanism fosters user-centric enhancements, ensuring that AI search engines grow alongside their user base.
By actively listening to users and adapting accordingly, AI search engines can achieve higher levels of user satisfaction and engagement, ultimately establishing a more trustworthy search environment.